Empower your Medical Imaging Solutions with our AI Modules
We enable medical imaging companies harness the power of artificial intelligence to enhance their existing products. With our expertise, we implement the latest of machine learning and image analysis technologies as backend modules with perfect and seamless integration. We own the experience of integrating them into systems in a way to maximize their value to our clients’ businesses.
Pick The Scheme That Fits Your Business
DEVELOPMENT OF AI-POWERED MODULES FOR MEDICAL IMAGE ANALYSIS AND QUANTIFICATION
Why Intixel?
- Data is secure with our anonymization tools
- Experience with wide spectrum of medical images (CT, MRI, PET, US, X-Ray, and Mammography)
- In-house GPU clusters for offline training
- Reliable warranty for model improvement
- Training partner-side developers to support long–term maintenance plan.
- Data labeling and annotation process support
- Experience with clinical data mining
- Support integration of our developed AI-Modules with both client- and server-side platforms
What can we do else?
Extraction of STRUCTURED DIAGNOSIS from Hand-written Reports
Extraction of labels/digital diagnosis from hand-written is a cumbersome task. So, we supply natural language processing (NLP)-based label extraction tools that obtain a digital and structured clinical indication from the hand-written medical diagnostic reports. such labels can be used to train AI-based predictive models.
Labeling and Annotation of Medical Images
Building an AI predictive model require training samples accompanied by its labels or annotations. Radiological data by nature are not accompanied by these digital labels or annotations. Therefore, we are supporting our clients with our in-house developed annotation and labeling tools. Our annotation tools are semi-automatic and validated by experienced clinicians which insure fast and accurate labels and annotations.
Why you need labels/annotations? To build artificial intelligence predictive models for medical images, you need a balanced and labeled dataset. This means that for each data point (in radiology, for each image) you need to define the ground truth. For example, if you want to build an algorithm that is able to differentiate between malign and benign tumors, the medical images in your dataset need to contain images with tumors and each image needs to have a label “benign” or “malign”. This will help the computer to learn how different tumor types can be recognized on the images.
Portfolio
Projects done by Intixel
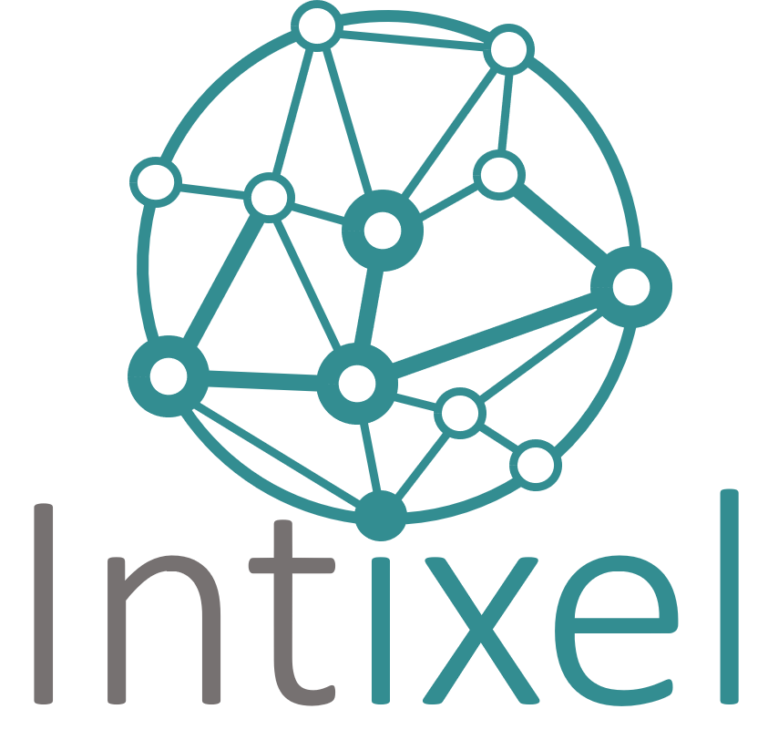
Cardiac MRI Segmentation
In partnership with Cardiac MRI Image Analysis Leader, segmentation of the left ventricle in Cardiac Strain-Encoded MRI images was successfully done and delivered as a seamless integrated module.


FFDM Tumor Localization
Intixel team has developed a deep learning-based screening module to one of the PACS vendors that classify Full-Field Digital Mammogram image that to normal and abnormal scans and segment abnormal breast tissue as an abnormality region of interest.
Skin Cancer Detection
Moreover, Intixel team has developed a deep neural network model that can classify and segment skin lesions in Dermoscopic images with a very competitive accuracies.
